With the rise of emerging technologies and the anxiety to experiment with intelligent models, many companies are omitting key processes for their effective implementation.
We live in a time when data seems to rule the world. The feeling is that with a large enough data set, companies have at their fingertips the possibility of applying artificial intelligence (AI) and doing magic: launching products to raise millions of dollars, generating unforgettable experiences for their customers or taking efficiency to a superlative level.
The reality is that accumulating data is only part of the job, but not the most important. How they are used, for what purpose, how safe they are, who uses them… Companies that don’t answer all those questions before embarking on an AI project could run up against ordinary natural stupidity.
To truly capitalize on the power of AI, you need to do the less glamorous work tied to the adoption of the most emerging and disruptive technologies first: getting data ready. This is where the concept of data governance comes into play: the set of policies, processes, practices, tools, methodologies, and people to ensure that data is available, of quality, and treated with the highest levels of privacy and security.
“Although the implementation of data governance programs in Argentine companies is still incipient, they have undergone a functional and timidly technical evolution,” says Daniel Menal, director of data and analytics for NTT DATA Argentina. The company proposes a maturity framework that considers the data governance office, master data and quality, lifecycle, architecture, security, linked services, communication, and literacy as measurement areas. From there, he found that most companies in the country oscillate between levels 2 and 3. “Having state-of-the-art technology without a solid data literacy strategy is not sustainable over time,” he concludes.

And it’s not all about artificial intelligence. Data governance is the key to any digital initiative going forward. Carlos Christian Sueiro, lawyer and professor of computer crime at the University of Buenos Aires, reminds us: “We often arrive at the false belief that only AI drives the fourth industrial revolution, but in reality it is the convergence of this with other exponential technologies such as biotechnology (gene editing, artificial DNA, pluripotent cells, chimerization) nanotechnology (miniaturization of elements at the nanometric scale), infotechnology (robotics, automation in general) and cognotechnology (neurosciences and neurotechnologies)”.
Ensuring Governance
“The issue of data governance in Argentina is in a process of evolution and is presented as a key tool to ensure the quality of data, but it still faces several challenges to reach greater maturity,” says Lautaro Carmona, CEO of Unitech, which specializes in digital solutions for the judicial sector. “Achieving this requires a complex organizational transformation, a sustained commitment over time, and political support from executive management,” he says.
Martín Malievac, director of research and development at Napse, which specialises in omnichannel solutions for retail, agrees that “there is still a long way to go for it to reach greater maturity in Argentina, but technological progress in the country is constant”. In addition, he states that the key to moving towards new stages is “to have a comprehensive and coordinated approach that promotes, in the first instance, greater investment in processes and technologies that make possible higher levels of traceability, coordination, exchange and security in the processing of data”.
This is not an issue that is the sole responsibility of the big companies. “The concept of data governance is often associated with complex structures that larger companies or those whose product or service depends on careful data processing must worry about, but the reality is quite far from it: establishing a data governance structure, policies and strategy early on is critical, not only to avoid high future costs, but also because by ensuring a higher quality of our data, we impact the efficiency, revenue and security of our users,” says Kevin Hansen, data science manager at Smart Channel, a self-managed predictive marketing platform.
In the age of the cloud, the solution is many times closer than you think. “More and more companies are opting for cloud platforms with components such as tools to evaluate data, catalog metadata, manage access control or monitor the quality and security of information,” says Natalia Scaliter, general manager of Google Cloud Argentina. “Protection, compliance with regulations related to data custody, and data control and visibility are key factors at this point,” he observes.
Let it be law
Paula Angeleri, director of quality at the University of Belgrano (UB), recalls that the Undersecretary of Information Technologies of the Chief of the Cabinet of Ministers issued a provision last year (2/2023) that includes an annex with recommendations for reliable IA.
“Although it promotes improvement, there is still a big problem to be solved: the lack of integrability between the different databases of the public sphere at the provincial and national level.” The UB collaborated with a group of government and academic leaders and IRAM to develop a new integrability standard (IRAM 17610-1). He also developed the MyFEPS framework, in Spanish and free to use, whose QSAT quality model (an acronym for “Quality” plus the surname of its three creators, Amos Sorgen, Angeleri herself and Rolando Titiosky) includes the aspects of data quality and security.
“Current legislation needs to be updated to meet the technological challenges related to privacy,” says Nahuel Alberti, head of Engineering at Paisanos, a creator of innovative digital products. “For greater maturity in data governance, it is crucial to adopt a comprehensive approach that ranges from legislative updates, such as the Personal Data Protection Act, the promotion of a data-driven culture in the public sphere, investment in technology and training.”
Sebastián Pereira, CIO of AI specialist Santex, highlights some challenges related to infrastructure and political or legal issues. “A central problem is the legal location of companies, which are often registered outside the national territory, and the location of servers in foreign territories,” he says.
“This complicates the enforcement of local laws and the protection of users’ rights due to the lack of jurisdiction,” he adds. On the other hand, it ensures that insufficient investment in protected and dynamic data ecosystems persists. “The evolution towards a balance that encourages autonomous development and not just the consumption of technology is crucial, including access to databases for model training and information sources that allow companies to innovate with legal certainty and obtain benefits in a multidimensional way.”
Driving Intelligence
Data governance is the necessary and sufficient step to achieve optimal results with AI initiatives. It’s the foundation of any data project,” says Menal.
At this point there is no crack. All those consulted express themselves in the same direction. “Poor data management could slow down the development of AI projects when there are difficulties in ensuring data security and privacy,” explains Pablo Velan, CTO of Argentine fintech N5. “It’s vital to understand the origin, sensitivity, and lifecycle of the data we use to power AI. There is no way to implement a successful project if a company, regardless of its industry, is not up to the highest standards of data management,” he adds.
“Effective data management in terms of quality, availability and usability is critical,” says Aldo Tirapegui, SAP Business Technology Platform Solutions Advisor for the Southern Region. On the first point, he says that “it is important to establish reliable processes to guarantee the quality of the data: implementation of cleaning procedures, standardization and validation.”
Regarding availability, he adds that “companies can use integration capabilities to combine data from different sources in one place and ensure that it is usable for analytics teams and AI projects.” Finally, in relation to accessibility, he indicates that “analytics solutions allow you to create interactive dashboards and visualizations that facilitate exploration and analysis.”
“Adopting a rigorous approach to data governance will allow us to dismantle the silos that limit the richness of the analysis provided by technologies such as generative AI and, in this way, make more accurate and timely decisions, in addition to improving expense controls by managing resources effectively, for example, being able to eliminate duplicate data,” says Scaliter. Google Cloud estimates that by 2025 all analytics systems will be powered by generative AI.
What can go wrong?
Observing with the inverted lens… What are the harms that can be suffered by a company that moves forward with its AI initiatives without having established good data governance?” Among the negative and far-reaching consequences of poor data governance are the possibility of giving rise to biases and wrong decisions, and a failure in data protection can result in privacy violations that lead to a loss of trust and legal sanctions that affect the viability of projects and a loss of trust in people about how AI can change our society for the better,” says Alberti.
“If the data is of low quality or biased, AI models can generate incorrect or unfair results, leading to decisions that harm certain groups or individuals and damage the public’s trust in the technology,” adds Matías Nahón, general manager of Analitix, a consulting firm specializing in corporate fraud. “It can also happen that incomplete or incorrect data reduces the accuracy of the models and their effectiveness in making predictions or making automatic decisions,” he adds.
“Not only does it involve operational and legal risks for a company, but it can also have a direct and significant impact on its market valuation,” Pereira details.
“Let’s think about a dataset of which we don’t know its origin, the processes involved for its generation and storage, and that doesn’t have a person in charge at a business or technical level… How could we trust AI-generated content from that?” asks Matias Salmeri, head of data for Argentina at the software factory Endava.
The person in charge
You can’t make a good government without a good team. A mature governance program necessarily involves the definition of new roles in the organization.
“Within the best practices in data governance, it is essential to have a data governance office (DMO) that ensures the implementation, monitoring and continuous improvement of the policies, processes, technologies and people associated with working with data,” says Menal.
Sergio Aguilera, director of computer science careers at the University of Belgrano, cites the guide to data governance roles prepared by the Secretariat of Innovation and Digital Transformation that includes a CDO, a data champion, a business owner (or person responsible for quality and processes), a technical owner (or responsible for data repository systems), a business custodian or expert, a technical custodian, and a data user. “The same guidance clarifies that not all organizations should have all roles, while recommending an information security custodian independent of technical custody,” explains Aguilera.
The market is starting to feel the pressure. “In recent years, searches for positions related to the use and management of data have grown exponentially,” says Daniel Iriarte, associate director of Glue Executive Search, a boutique executive search and top management consultancy. “The chief data officer (CDO), with responsibility for protecting information privacy and data management, is one of the most sought-after profiles with the greatest projection for the coming years,” he clarifies.
However, he assures that it is not easy to hire them. “They are not abundant in the market and, in addition, Argentine companies must compete with offers from abroad.” And he says that the professionals who occupy these positions will need to incorporate knowledge of “data brokering, to generate commercial agreements between data companies and those who buy fragments of data”.
Roles, roles, and more roles
There is no one position more essential than another, they are all necessary within this new logic that brings about a new way of business and technology.
“Also noteworthy is the data steward, which is integrally responsible for a particular set of data (there are usually as many as there are domains of information in the company) and a bridge between the business and IT, and the data custodian, the technical counterpart of the data steward, the guardian of access, movement and storage,” says Salmeri.
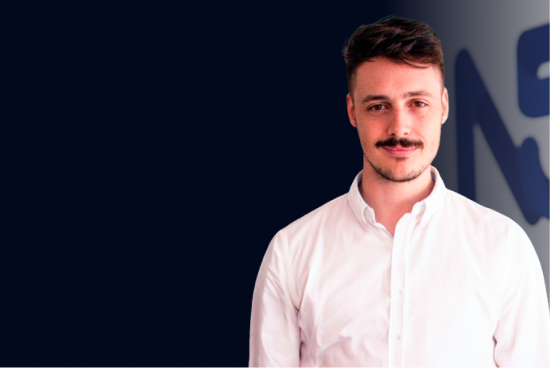
These functions should not be seen as isolated events. “It requires a combination of these, which in turn need to work together to build a strong data culture,” says Velan.
Carmona also mentions the presence of lawyers on the team. “Structured as a committee, it is often essential to ensure protection throughout the entire lifecycle, from the creation of the digital asset to its destruction,” he clarifies.
Alberti adds the data ethicist, “which is responsible for ensuring that the use of data is in line with ethical and responsible principles, especially in contexts where personal and sensitive information is used.
“Something to note about governance positions is that they must have a solid technical knowledge base, but it is just as important that they have process engineering knowledge and skills such as leadership, communication and negotiation, as they act as bridges between technical and non-technical areas,” says Hansen.
We live in a time when data seems to rule the world. But first, companies that want to capitalize on this situation need to govern data.
Editorial: El Cronista