IT IS ESTIMATED THAT BETWEEN 3% AND 27% OF CHATBOTS’ ANSWERS ARE WRONG. BY FOLLOWING A SERIES OF TIPS, THE MARGIN OF ERROR CAN BE REDUCED TO A MINIMUM.
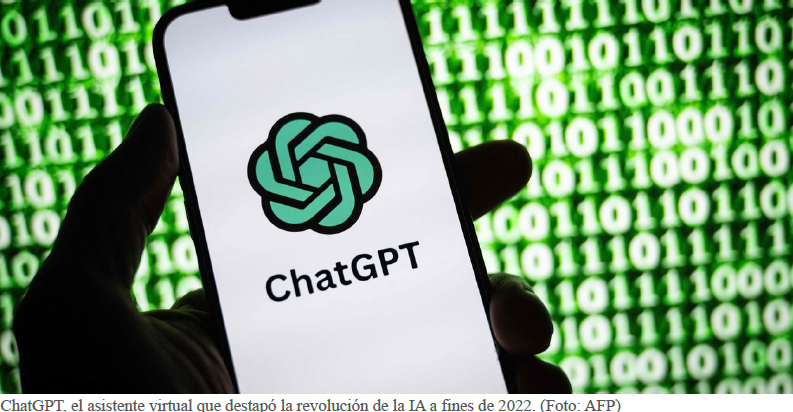
Generative artificial intelligence (AI) chatbots are not infallible: they make mistakes that often go beyond providing false information. Specialists conclude that these systems are programmed in a way that can lead them to “hallucinate.”
Generative AI is here to stay in the daily lives of people and companies, so much so that in the fintech world it was already valued at 1.12 trillion dollars in 2023. The rapid growth of its rates suggests that it will reach 4.37 trillion dollars by 2027, according to a Market.us report. Like any tool in full evolution and growth, AI will also have to face different barriers and problems
WHAT ARE ARTIFICIAL INTELLIGENCE HALLUCINATIONS?
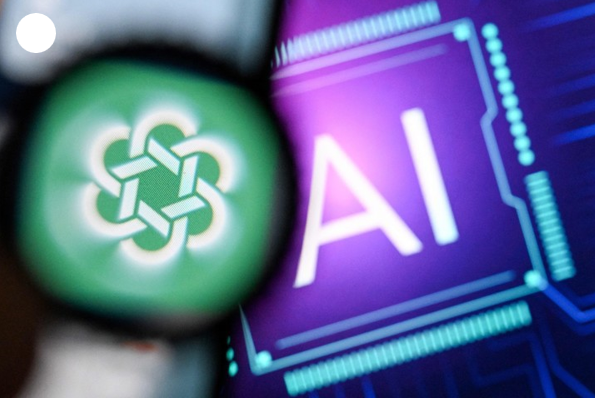
Algorithmists refer to incorrect or misleading results produced by AI models – ChatGPT, Llama or Gemini – as “hallucinations”. These failures can be due to a variety of factors, such as insufficient training data, incorrect assumptions or biases in the data used to train the model.
Hallucinations are a serious problem for AI systems used to make important decisions, such as medical diagnoses or financial trading.
Models are trained on data and learn to make predictions by looking for patterns. However, the accuracy of these predictions often depends on the quality and integrity of the data used to train them. If they are incomplete, biased or flawed, the AI model can learn incorrect patterns, leading to inaccurate predictions or hallucinations.
For example, an AI model trained on medical images can learn to identify cancer cells. However, if there are no images of healthy tissue in that dataset, the AI model could incorrectly predict that the healthy tissue is cancer.
“Models are trained with large (very large) volumes of information, and from there they learn patterns and associations with that information that may still be insufficient, outdated or inconsistent, and since they have no ‘understanding’, they don’t really know what they are saying,” explains Pablo Velan, CTO and partner at N5, a software company specializing in the financial industry.
According to analysis by startup Vectara, the hallucination rate of chatbots ranges from 3% to 27%.
Bad training data is just one reason why hallucinations can occur with AI. Another factor that can contribute is a lack of proper foundation.
An AI model may have difficulty accurately understanding real-world knowledge, physical properties or factual information. This lack of foundation can cause the model to generate results that, while seeming plausible, are actually incorrect, irrelevant or meaningless.
Along these lines, the manager details that when faced with ambiguous questions or without sufficient context, the AI’s ability to interpolate and extrapolate to respond comes into play and will make incorrect generalizations or assumptions.
This situation may not be a concern for those who use the tools for mere fun. However, it can generate major problems for companies where precise decisions are crucial.
Likewise, Velan reflects on whether there is a “cure” and points out that there are algorithms that avoid this type of error by not being connected to the Internet, since they are trained within closed sources of information, which is why they are trained only with data from a particular entity.
On the other hand, IBM specialists identified that AI models could follow certain steps to reduce these false perceptions and make the systems work optimally.
TIPS TO AVOID HALLUCINATIONS IN ARTIFICIAL INTELLIGENCE
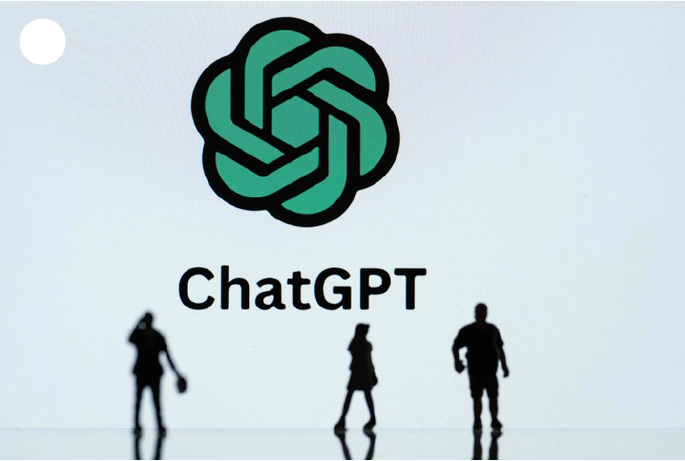
Define the purpose of the AI model
Explaining how the AI model will be used (as well as the limitations on using the model) will help reduce hallucinations. To do so, a team or organization should establish the responsibilities and limitations of the chosen AI system; this will help the system complete tasks more effectively and minimize irrelevant and “hallucinatory” results.
Use data templates
Data templates provide teams with a predefined format, which increases the likelihood that an AI model will produce results that fit prescribed guidelines. Relying on this solution ensures consistency of results and reduces the likelihood that the model will produce erroneous results.
Limit responses
AI models often hallucinate because they lack constraints that limit possible outcomes. To avoid this problem and improve the overall consistency and accuracy of results, define boundaries for AI models using clear probabilistic thresholds or filtering tools.
Continuously test and refine the system
To avoid hallucinations, it is critical to rigorously test the AI model before use, as well as continuously evaluate it. These processes improve the overall performance of the system and allow users to adjust or retrain the model as data ages and evolves.
Rely on human oversight
Ensuring that a human validates and reviews the AI’s output is a final containment measure to prevent hallucinations. Their involvement ensures that if the AI hallucinates, someone will be available to filter and correct it.
A human reviewer can also offer subject matter expertise, improving their ability to assess the accuracy and relevance of the AI’s content to a given task.
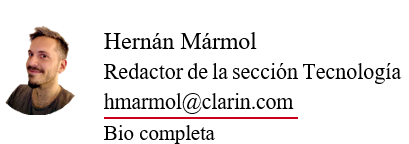